Business Modeling: Getting it Right
Best-selling author Atul Gawande once broke mistakes down to two broad categories: mistakes of ignorance and mistakes of negligence. When contemplating a major decision or transaction, businesses spend vast amounts of time and money focusing on the former. They then trust that by hiring responsible people giving their best effort, they will be able to avoid any mistakes of negligence. On the face of it, that makes sense: it should a lot harder to know the unknown than to make sure that you aren’t adding when you should be subtracting. Why then, do we all have stories of models and analyses gone wrong? More importantly, how do we prevent the mistakes of negligence that created these stories?
The Uncomfortable Truth About Models
When you take a step back and think about it, the fact that models go wrong so often is understandable. The fact is that even well-trained and attentive modelers will make mistakes. Studies on human error rates have shown errors ranging from 0.5% to 10.0% with the general rate for more complicated tasks falling at about 5.0%[1]. An average deal model contains about 10,000 unique formulae. Even if you use the low end of the range of 0.5%, that’s 50 mistakes in your deal model’s code. That is simply not good enough.
You also should consider the hundreds of assumptions that often feed into models. There’s always the risk that someone inputs a negative when it should be a positive or enters an annual figure into a monthly input. Again, normal human error rates multiplied across a large number of inputs yields a result that is simply unacceptable. However, there is a more insidious and important issue at play in most models: ignored relationships between assumptions.
Considering relationships between assumptions can be tricky. If you have two assumptions in a model, then all you need to do is make sure that assumption A does not have an impact on assumption B and you are free to model as you please. That’s easy enough. However, as you expand the number of assumptions, the number of relationships you must consider expands exponentially. By the time you’ve reached 50 assumptions, you have 1,225 relationships to consider. If you have 500 assumptions, you are looking at 124,750 relationships. The vast majority of relationships can be summed up simply with “no material relationship” but not all of them can be viewed this way. It takes a lot of care to make sure that you aren’t assuming “no relationship” when the correct answer is “it’s complicated.”
The Devil is in the Details
I once advised a client who was considering entering into an agreement with a partially owned subsidiary. It was a complicated transaction and they had gone to great lengths to try to make sure that they had their bases covered. When I asked them about customer attrition, they said something to the effect of, “There isn’t much attrition data so we were conservative and assumed 20% attrition, so 80% of year 1 revenues are from existing customers, 64% of year 2 revenues are from existing customers, etc.”
That would have made sense had they not also considered 20% revenue growth. If you do the math, that’s not 20% attrition; it’s 4% attrition. No matter how they looked at the numbers, a 4% attrition number was far too low. This mattered. The deal was structured around different payouts for existing and new customers. By changing the attrition assumption, the deal went from a great one to a financial catastrophe.
Five Recommendations for a robust model:
1. Get a fresh pair of eyes, but make sure they’re the right eyes
It always helps to have someone new come in and go through a model. After a certain point, a model’s developer is just too well acquainted with the analysis to spot seemingly obvious mistakes. A new reviewer can bring a fresh perspective and greatly increase the likelihood that errors will be spotted. Often, this is referred to as a “math check” and is delegated to low level employee or an offshore resource. While helpful, this is a wasted opportunity to not only check the “math” but also catch some of the deeper more difficult logic-related errors that may be flowing through the model. Before sending a model to someone to check, ask yourself, “Does this person actually understand the business and the problem I am trying to solve?” If the answer is no, you should consider finding someone else.
2. Understand the assumptions and their impact
At A&M, we developed a tool that cycles through every assumption in our models and shows the impact on pre-selected outputs. This does two things: a) allows us to see what assumptions have the most impact on the model and b) allows us to quickly ensure that each assumption is moving the model in the way we expect. The first task allows us to focus on making sure that we are focused on the most material assumptions. Think of the example above, if the client had done this, they would have known the impact of attrition and would have given a lot more thought towards it. The second task allows us to quickly spot errors in both inputs and calculations. If an increase in the cost of sales results in an increase the Internal Rate of Return, something has gone wrong. Just by experimenting with your assumptions and seeing how they move the end numbers, you can learn a lot from a model.
3. Give yourself time to test
Models can be like gas - they expand to fill the space in which they are allocated. Far too often, a two-week analysis turns into one week and six days of model development, adding scenarios, sensitivities, new outputs and dashboards followed by a few hours of hurried checking and error catching. To avoid this, you need to understand the end goal, and work efficiently and effectively towards that goal. You also need to understand what contributes to the goal and what is just “modeling for modeling’s sake.” Getting those two components right will help you allocate an appropriate time towards testing and ease the risk of error.
4. Value simplicity, particularly when structuring and coding models
We often like to say that models should be readable and they should read like boring text books, not spy thrillers. When building a model, it pays to take an extra few rows to break calculations down into individual explicit components rather than grouping them together into one big long formula. Through structure and labeling, developers should be able to walk through exactly what their model is doing without showing any of the formulae.
When we run model reports, which show us model structure, formula used, etc., the first thing that I typically look for is the most complicated formulae. I don’t look at them because they are more likely to be wrong. In fact, modelers often focus so much on the most complicated stuff that it’s surprisingly unlikely to find a mistake there. The reason why I focus on the most complicated formulae is to determine if they had to be so complicated. If the answer is no, then I can be fairly certain that there will be mistakes in the model.
5. Recalculate and reconcile
When modeling, if there are two paths to a given destination, it’s often a good idea to take both. For example, models often calculate sales forecasts on a percentage growth basis or based on forecasted price times forecasted based on quantity. Conducting one type of forecast and then recalculating based on the other method will allow you to easily compare and ensure that you haven’t made a mistake, which is helpful on its own. However, there are much wider benefits to doing this. It will also allow you to examine the implicit assumptions in your forecasts. We consistently see clients confidently apply overall growth forecasts and then struggle to describe whether the growth will be driven by higher prices or higher volumes. On the other hand, it’s not uncommon to see an analysis put in detailed price and quantity figures that arrive in an unsupportable overall growth forecast.
Before acting on the outputs of a model, remember to ask yourself:
- Have I given the right person or people enough time to ensure that this is functioning as intended?
- Is this model actually doing what it intends to do?
- Do the key drivers of the model have any relationships that the modeler may have missed?
You can never reduce the risk of modeling errors to zero. While diligence, skill and adherence to best practices can help you bring that risk down, it won’t be enough. In order to reduce your risk to an acceptable level, you have to be keenly aware of the types of risk you are facing when modeling and actively manage them through effective processes and review.
Relationships to variables
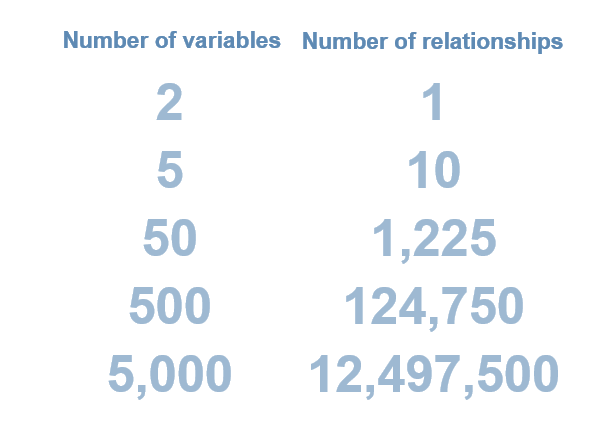
Estimated mistakes:
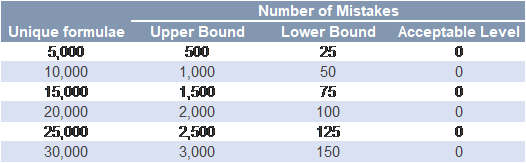
Client example:

About the Author
Devin Rochford is a Director with Alvarez & Marsal in New York where he specializes in Business Modeling & Analytics. Devin has over 10 years of experience as an advisor where he has delivered solutions to clients in a broad spectrum of contexts ranging from merger and acquisition (M&A) advisory, research and development (R&D) licensing strategy, and setting the draft structure for a leading cricket league. Read more.
[1] http://panko.shidler.hawaii.edu/HumanErr/Basic.htm