Understanding the Differences Between Structured and Unstructured Data in Disputes
Introduction
When we think about what type of data would be important in a dispute, we are immediately drawn to the usual suspects: emails, user generated office documents and paper. They are all examples of unstructured data, which makes up between 40 percent and 80 percent[1] of all data in an organisation and on average is growing up to 23 percent annually[2]. Although dispute cases mainly focus on unstructured data, structured data can be of equal importance to help understand an organisation’s corporate environment.
Structured v. unstructured data - what's the difference?
Structured data refers to information stored in fixed fields that is comprised of defined data types such as dates, numbers and text. Characteristics of structured data include: easy to store, query and analyse. Some common examples of structured data include data contained in relational databases and spreadsheets, such as account payable data and payroll.
The content of unstructured data, on the other hand, has no identifiable structure (although some data types do have an associated structure like emails with to, from, cc fields, etc.) and can't be so easily analysed, as it is not organised in a way that allows the straightforward identification of meaningful relationships between data elements.
Why is data important to an organisation?
Data is a metric of every aspect of an organisation and forms the backbone of its business processes. When we start planning for a dispute, we shouldn't limit ourselves to either unstructured or structured data, but rather focus on how different data sources can be used to complement each other.
Unstructured data is easily accessible, easier to understand and it gives us the information we need to build a picture of how an event or series of events occurred. We use it every day - almost all day. Take any given active project on any given day and there will be a plethora of emails, Microsoft Office documents and PDF scans containing project-related information, existing in two main places: emails and a network location. By comparison, structured data requires an understanding of multiple business systems and query language to enable relevant information to be identified and extracted. Consider now the structured data that will be tracked on a typical project and ask your self - what billable hours have been recorded against that project? What interactions have been recorded in the customer relationship management system? What expenses have been entered into the expense and travel system?
When it comes to the running of the business, it's the structured data sets that contain the detailed day to day information we are interested in, which can provide the supporting information required in a dispute. The true value in working with structured data lies in the power of being able to perform complex data analysis over hundreds of millions of transactions in a short period of time. Structured data allows us to build a complete and accurate picture of an organisation from the bottom up, which can then be combined with the top down view that unstructured data gives to provide a holistic view of an organisation. High level figures in reports or financial statements can provide a one-sided view, and by losing details caused by aggregation or changes over time, it might not show the full story. By delving into the underlying transactional level information and performing independent calculations, the exceptions, errors and inconsistencies become clear.
What is the value of structured data in disputes?
There are many types of disputes where structured data can be used to support and test hypotheses. Because structured data feeds into management information systems that allow key stakeholders to make business decisions, as well as enable finance teams to leverage finance systems to calculate year end results, and HR systems to monitor staff turnover and job applications, it can provide valuable insights and answer key questions critical to a dispute.
Some areas where structured data can be used to add value include:
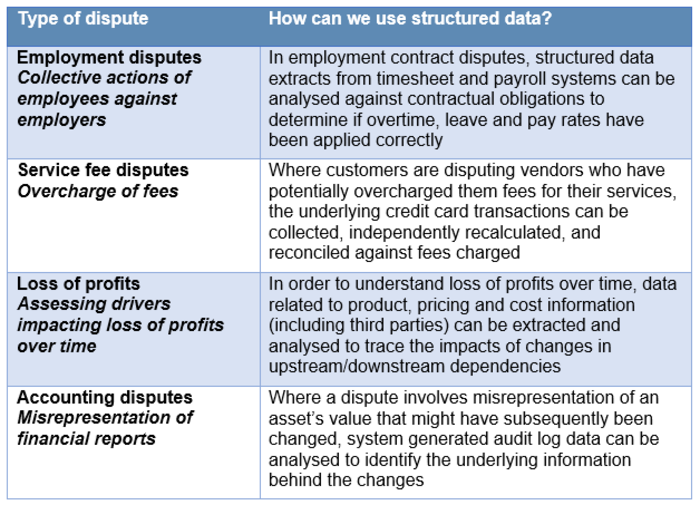
How can this data be obtained and used?
Now that we know it's important, how do we get it? The approach to working with structured data shares many similarities with the approach to working with unstructured data but there are some key differences.
There are typically five stages when working with structured data:
- Scope
- Extract
- Load
- Clean and Transform
- Insights and Reporting

Scope
The approach to working with structured data starts off the same as unstructured data. It is about identifying which systems contain the data that may be relevant to the dispute and determining the scope of the requirements. Data mapping helps build a picture of how an organisation’s business systems talk to each other and which individuals have access to what data. Prior to the extraction process, we need to preserve the data. An understanding of the way in which the business' systems store data and the backup processes that are in place is needed in order to successfully preserve data. Sometimes the live data won’t include the time period relevant to the dispute and access to backups will become important, as will ensuring they are not overwritten.
Extract
Just like with the collection of unstructured data, it’s imperative that structured data is collected using a defensible method, as efficiently and effectively as possible. Forensic tools and procedures are utilised to achieve this. As the data is usually large in volume, constantly changing and critical to the business, techniques such as mirroring are implemented to enable efficient and parallel processing of the data.
Load
The extracted data is loaded into an analysis engine, typically a database. As we might be bringing in data from multiple systems in several different formats, it is important to standardise the data and convert it to the same format to make it meaningful. There are several tools that can be used, depending on the type of data, but the key thing to consider is that whatever tool is used, it should ensure that the process is auditable, repeatable and robust.
Clean and transform
The data quality of the extracted data is then assessed, and data cleansing and transformation are performed to ensure consistency and accuracy of the datasets. White spaces are removed, dates are converted to a standard format, relationships between datasets are established and a reconciliation process is performed to ensure the completeness of the data. Once clean and transformed, the data from different sources is consolidated to allow quick and easy access and interrogation of data.
Insights and reporting
Depending on the nature of the dispute and the supporting data, several analytical tools can be utilised to allow users to perform exceptions testing, forecast modelling, valuations, reconciliations, and financial transactions testing, to mention a few. Reports can be automated for repeatability (e.g. weekly/quarterly reports). Various visualisation and reporting tools, like Tableau or Microsoft Excel can allow users to further drill down into output tables, and simple graphs and bespoke dashboards offer a greater capability for investigation with user-friendly dynamic interaction.
Common problems
As one would expect, understanding an organisation’s data environment can be tricky. Common issues you might encounter when working with structured data could include:
Poor quality data
It is important to cleanse and transform ‘dirty data’ to ensure consistency and accuracy of the datasets. This could involve standardisation of key categorical fields, testing for relationships across datasets, exclusion of certain records and reconciliation of detailed record totals against reported values.
Multiple systems in place with different formatted data
Structured data is often located across multiple business systems within an organisation that don’t necessarily talk to each other and don’t allow data to be exported in a consistent manner. To facilitate the analysis and review of this data, it must be exported and then combined into a data warehouse, giving users the ability to connect and query information from different parts of an organisation and gain actionable insights, or the ability to export data in a format that can be loaded into a review platform.
Change to a new system, resulting in differences in data extracts over time
When organisations migrate to a new business system, legacy systems are rarely fully decommissioned and are maintained for reporting to enable access to the legacy data. The issues with dealing with data that resides in both legacy systems and live systems are twofold; firstly, the employees who understand and administer the legacy systems may no longer work at the company, making identification and extraction more difficult, and secondly, the unique identifiers in the databases may have changed between the legacy and new systems. Key fields may no longer be used or renamed, new information may now be available and some data may exist in both systems and be duplicative. It is necessary to perform a deduplication and clean up exercise to link information and validate the data before performing any analysis.
Volume and complexity
It may seem small when compared with unstructured data but in relation to structured data, information is big in volume and complexity. As mentioned above, we can't just extract it and use it - it's not always user-friendly. We need to normalise, cleanse and consolidate it into one system first before we can gain insight into the data. No one wants to look at millions of accounting transactions in a spreadsheet, so it is necessary to provide the reporting and insight to identify anything that is relevant. There is also the issue of redundant data, as with the review of structured data, it is important to remove any duplicative data or anything unnecessary to the scope of the dispute.
Transparency of costs
It's become more straightforward to provide costs for collecting unstructured data. Comparatively, it's more difficult to predict the costs for collecting data from ERP systems where data is spread across tens or hundreds of database tables and we need to understand how the database tables relate to each other and how the system retrieves or provides information from / to other systems. Even after the collection, it will be necessary to recreate the database for the purpose of extracting, normalising, cleaning and presenting the information in a format that can be analysed or reviewed efficiently.
Case study
The following case study illustrates the kinds of requests A&M is seeing in relation to structured data in disputes.
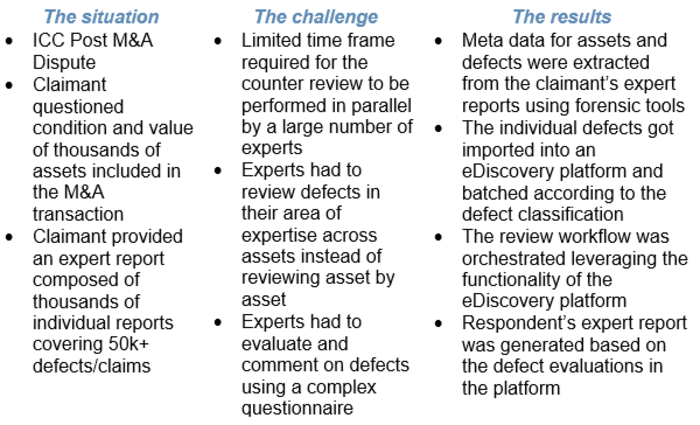
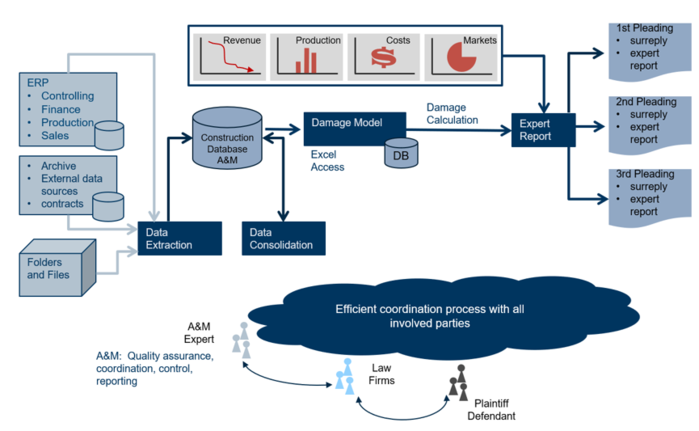
Conclusions
If we were not thinking about structured data before, we should be now. It flows through all business-critical processes from something as complex as an inventory management system or through a series of Microsoft Excel spreadsheets recording the same information. When it comes to disputes, valuable insights can be gained from the data that sits in these business applications. In this context, we refer to insights not driven purely by qualitative information or the analysis of small samples, but, for example, by testing complete populations of transactions and quantification of associated damages, providing concrete data-driven fact-based views of a case. By reviewing 100 percent of all records rather than only considering one aspect of a case, any analysis is more accurate.
We started off this paper by comparing structured data against unstructured data but when we start planning for a dispute, we shouldn't be thinking about them in isolation. They both have their merits in a dispute and they complement each other. It's easy to disregard structured data due to its perceived complexity and the expense to preserve and collect it, especially in an age where better transparency of costs is demanded, (one of the drivers for Practice Direction 51u was perceived excessive cost in disclosure) but ignoring it for these reasons can leave you without the necessary evidence. If you have the right people with the right experience, you should be able to confidently implement an efficient workflow and predict the associated costs.
[1] https://www.dataversity.net/unstructured-data-everything-your-company-should-know-about-it/
[2] https://www.datanami.com/2019/01/31/data-management-falls-short-for-unstructured-data/