Identifying Cross-Functional Risk in a Global Environment
Companies that operate in multiple jurisdictions and possess complex structures, functions and processes across their value chain are challenged to sufficiently meet compliance requirements due to resource limitations, diverse processes and unwieldy organizational design[1]. Controlling and mitigating risk is even harder when there hasn’t been proper identification of potential sources of risks across people, products and locations. Think of the challenges in identifying compliance risk for an original equipment manufacturer (OEM), exporter or retailer that considers sourcing, transportation, manufacturing, distribution, marketing, sales and finance functions (just to name a few) across a global footprint. A company’s internal audit function, risk officers and management may be charged with applying limited resources to a seemingly never-ending catalogue of risks, certain to include fraud, bribery, corruption, money-laundering, sanctions avoidance, market manipulation, consumer protection and data protection. So, what is to be done in pointing risk control measures in the right direction to gain the most effectiveness, efficiency and defensibility across the organization?
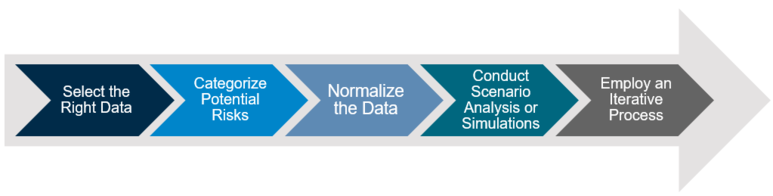
Select the Right Data
First, understanding your customers, vendors, methods of transport, money flows, material sources, employees, marketing and product quality controls across business functions is critical in identifying key risks. That understanding within large organizations is best attained by collecting and using suitable, reliable data that might help quantify risk exposure. Considerations in selecting the right data include:
- Building a preliminary risk catalogue
- Categorizing risks
- Understanding what data is available
- Determining if the data is relevant. Does is measure what it is supposed to measure?
- Determining if the data is unbiased. Are there indicators of selection, detection or reporting bias (among others) in the numbers and the collection approach?
- Identifying what data is not available, understanding why that is the case and how to obtain it.
- Is there enough quality data to appropriately address our preliminary risks and/or controls catalogue through data analysis?
In the case of assessing financial crime risk, the following examples of data types will be useful in painting a comprehensive risk picture.
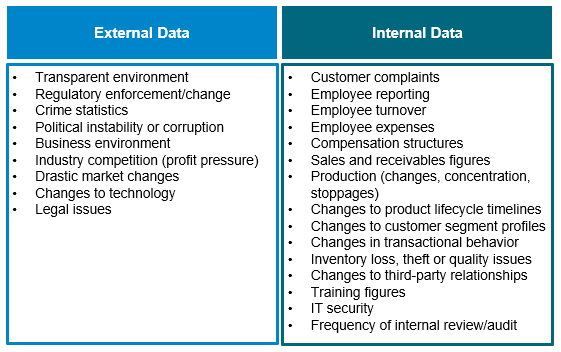
With our risk catalogue and data in hand, it is time to organize the data into as many categories, in accordance with our priority risk concerns (risk strategy). If we are assessing varying risk within the global organization, we might create the following risk categories using the Committee of Sponsoring Organizations of the Treadway Commission, Enterprise Risk Management — Integrated Framework[2]: Operations Risk, Empowerment Risk, Information Processing/Technology Risk, Integrity Risk, and Financial Risk[3]. We would then categorize each data set, ensuring certain categories are not over or under-represented in a way that could skew simulation results towards a particular category. This is potentially the most challenging aspect of the analysis, as data is not always uniformly available or reliable, so we are left to defer to, again, expert judgement in balancing and interpreting our categories (though underrepresentation of data may itself indicate a lack of risk controls in a particular category).
Normalize the Data
Now that we have developed a menu of data points and related categories, it is unlikely that the data is easily compared in a meaningful way. Certainly, running correlation tests between data sets can provide useful insights, but for a wider understanding of the areas and likelihood that risk may present itself across the organization, we’ll need to ensure we are comparing apples to apples… to apples… to apples. The disparate data (raw numbers, ratios, rankings, etc.) will force us to adjust values measured on different scales to a common calibration in order to properly compare and simulate risk outcomes. Simple ways of normalizing the data include rank-ordering or calculating percentiles within all of the data sets and maintaining consistency for what each data point means with regards to risk. For example, if we determine that a higher concentration of sales results in higher risk, we need to define if the higher rank-order or percentile number is interpreted as higher risk (or the inverse) consistently across other data points. Normalizing the data also mitigates risk of skewing final risk.
Conduct Scenario Analysis or Simulations
Hopefully, you have already engaged your organization’s statistics expert to assist in normalizing and manipulating the data with the aim of arriving at meaningful conclusions about your risk. Simulation models frequently include mitigating and contributing factors to assess the likelihood of risks occurring at specific places or during specific times. They are limited in that they cannot provide certainty of the likelihood of the real-world occurrences they aim to represent[4]. Model risk emanates from several sources, including:
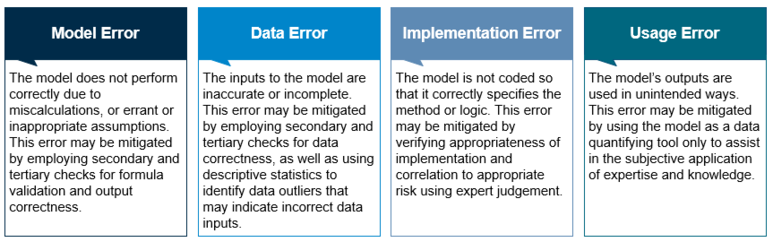
Some models to consider when combining and analyzing your data include probability distribution, sensitivity analysis, Monte Carlo analysis or tornado diagrams. Another simulation technique that may point towards risk concentration within a particular business function or geography, consists of arranging each of your data points into categories along an X-axis, and (rank or percentile) ordering them across geographies or business functions (Y-axis). After rank-ordering each category then simulating the Y-axis risk score for each permutation, we are able to estimate a total risk score (the sum of each score across every permutation) and use other statistical methods to arrive at score dispersion, threshold of statistical significance and “top-X” frequency. Again, this type of analysis is simply a tool for use in combination with other independent review, subjective analysis and expert judgement.
Employ an Iterative Process
Because statistical models and data are imperfect, conducting data simulations to detect risk over time is more likely to result in meaningful and actionable outputs. There are a few reasons why performing a methodologically consistent risk analysis on a semiannual or annual basis increases the effectiveness of the analysis including:
- New or updated data may confirm or deny previous model outputs.
- Drastic changes in model outputs can indicate changes in actual risk or model/implementation error.
- Changes in output may be correlated to changes in compliance program controls (good or bad).
- Consistent analysis can facilitate documented support and reasoning for compliance program updates and application of limited resources.
Conclusion
Compliance becomes more challenging as companies become larger, more dynamic and move into new markets. The risks that present themselves due to environmental factors, gaps in compliance organization, communication processes, controls, as well as testing and validation functions are more likely to be identified through effective data collection and analysis. Deriving meaning from that analysis is key to implementing prevention, detection and reporting initiatives to close those gaps that may result in financial, operational, reputational and regulatory risk.
To review a demo of A&M Financial Crimes’ risk simulation approach, please contact the author, Michael Carter at mcarter@alvarezandmarsal.
[1] Graham L, Bedard JC, Dutta S. Managing group audit risk in a multicomponent audit setting. Int J Audit. 2018;22:40–54. https://doi.org/10.1111/ijau.12103
[3] The risk categorization process is subjective and relies heavily on expert judgment. For this example, we chose COSO’s “Process Risks” since the data is likely indicative of process controls absence or failure.
[4] http://www.scientificpapers.org/wp-content/files/07_Dumbrava_Iacob-USING_PROBABILITY__IMPACT_MATRIX_IN__ANALYSIS_AND_RISK_ASSESSMENT_PROJECTS.pdf